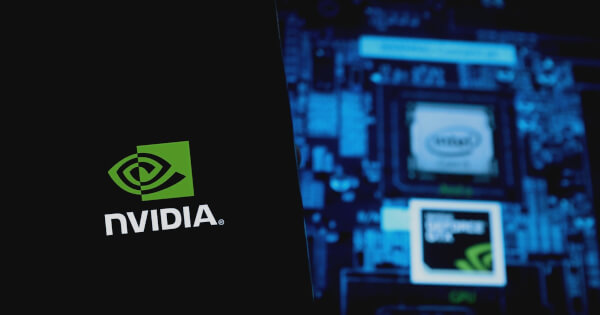
NVIDIA has set new performance and scale records in the generative AI domain, according to a recent submission to MLPerf Training v4.0. This achievement underscores the company’s ongoing dominance in AI training benchmarks, particularly in the realm of large language models (LLMs) and generative AI.
MLPerf Training v4.0 Updates
MLPerf Training, developed by the MLCommons consortium, is the industry-standard benchmark for evaluating end-to-end AI training performance. The latest version, v4.0, introduced two new tests to reflect popular industry workloads. The first test measures the fine-tuning speed of Llama 2 70B using the low-rank adaptation (LoRA) technique. The second test focuses on graph neural network (GNN) training, based on an implementation of the relational graph attention network (RGAT).
The updated test suite includes a variety of workloads such as LLM pre-training (GPT-3 175B), LLM fine-tuning (Llama 2 70B with LoRA), text-to-image (Stable Diffusion v2), and several others, covering a wide range of AI applications.
NVIDIA’s Record-Breaking Performance
In the latest MLPerf Training round, NVIDIA achieved remarkable performance using a full stack of its hardware and software solutions:
- NVIDIA Hopper GPUs
- Fourth-generation NVLink interconnect with third-generation NVSwitch chip
- NVIDIA Quantum-2 InfiniBand networking
- An optimized NVIDIA software stack
These components have been further optimized since the last round, enabling NVIDIA to break previous records. For instance, NVIDIA improved its GPT-3 175B training time from 10.9 minutes using 3,584 H100 GPUs to just 3.4 minutes using 11,616 H100 GPUs, demonstrating near-linear performance scaling.
Generative AI and LLM Fine-Tuning
NVIDIA also set new records in LLM fine-tuning, particularly with the Llama 2 70B model developed by Meta. Utilizing the LoRA technique, a single DGX H100 with eight H100 GPUs completed the fine-tuning in just over 28 minutes. The NVIDIA H200 Tensor Core GPU further reduced this time to 24.7 minutes. NVIDIA’s submissions also showcased scalability, achieving a fine-tuning time of just 1.5 minutes using 1,024 H100 GPUs.
The company leveraged the context parallelism capability available in the NVIDIA NeMo framework to achieve these results. Additionally, the use of FP8 implementation of self-attention in cuDNN improved performance by 15% at the 8-GPU scale.
Advancements in Visual Generative AI
MLPerf Training v4.0 also includes a benchmark for text-to-image generative AI based on Stable Diffusion v2. NVIDIA’s submissions delivered up to 80% more performance at the same scales through extensive software enhancements, such as the use of full-iteration CUDA Graphs and an optimized distributed optimizer for Stable Diffusion.
Graph Neural Network Training
NVIDIA set new records in GNN training as well. Using 8, 64, and 512 H100 GPUs, the company achieved a record time of just 1.1 minutes in the largest-scale configuration. The use of eight H200 Tensor Core GPUs provided a 47% boost compared to the H100 submission at the same scale.
Key Takeaways
NVIDIA continues to lead in AI training performance, showcasing the highest versatility and efficiency across a range of AI workloads. The company’s ongoing optimization of its software stack ensures more performance per GPU, reducing training costs and enabling the training of more demanding models.
Looking ahead, the NVIDIA Blackwell platform, announced at GTC 2024, promises to democratize trillion-parameter AI, delivering up to 30x faster real-time trillion-parameter inference and up to 4x faster trillion-parameter training compared to NVIDIA Hopper GPUs.
For more detailed information, visit the NVIDIA Technical Blog.
Image source: Shutterstock
. . .
Tags